Automated vehicles are a classic tease of technology. We’ve been told for years that they are just around the corner, but the vision keeps getting pushed back. Yet researchers are making progress to solve the core technology hurdles that will allow AVs to hit the roads en masse in the next decade or two.
That’s the view of Henry Liu, new director of Mcity, the University of Michigan’s public-private research partnership devoted to future mobility. Liu explores the intersection between transportation engineering and artificial intelligence. That expertise makes him a key player in shaping AV development: Mcity is perhaps best known for is a unique test facility for connected and automated vehicles, with more than 16 acres of roads and traffic infrastructure to simulate urban and suburban streets, and 5G-connected sensors to collect driving data.
That test track is invaluable to Liu and Mcity’s partners – like the Michigan Department of Transportation and the National Science Foundation – as it helps to develop sophisticated decision-making technology for AVs. The key to crossing the tech over the finish line, Liu says, is getting machine learning to account for the absolute rarest scenarios on the road.
“I’m confident that AVs will be in mass production in the foreseeable future, not 100 years later,” Liu says. “Somewhere around 10 to 20 years, we’ll be there.”
Liu discussed what challenges remain with Andreas Nienhaus, head of the Oliver Wyman Forum’s Mobility initiative, and Alan Wilkinson, lead partner for Oliver Wyman’s automotive practice in North America.
What are the bottlenecks to deploying AVs on public roads?
The key bottleneck is still the technology, but regulatory directives are also needed.
We want to be the voice of reason in setting AV policy, and it’s a key issue. The industry hinges on federal policy, and the lack of a federal framework on connected vehicles is causing a bottleneck for deployment and commercialization. It’s like the seatbelt – without the federal mandate, I don’t think seatbelts would be here today.
That lack of guidance affects consumer doubts about AV safety, too. Human drivers need to pass a vision and knowledge test, but no such thing yet exists for computer drivers. And it really should be an intelligence test, not a safety test for the computers.
How is Mcity helping to develop frameworks for AV testing?
We developed the testing in two stages. The first is scenario-based testing. We test specific functionalities of AV systems like whether you can do a lane change, an unprotected left turn, or merge from a ramp. That’s a behavior competency test more specific to certain scenarios.
The second stage of testing is more comprehensive. We take these AVs and let them run at the test facility. At the same time, we generate challenging scenarios constantly and see how the AV reacts to them. For example, within Mcity there are a few intersections. The scenario could be the traffic light turns yellow and there’s a pedestrian walking along the crosswalk and maybe another vehicle in front of the AV, blocking a line of sight. We can synchronize and design the scenarios. This is more like a road test for human drivers.
We’re hoping that this framework will serve as a blueprint for federal agencies to develop their own regulations in terms of AV testing.
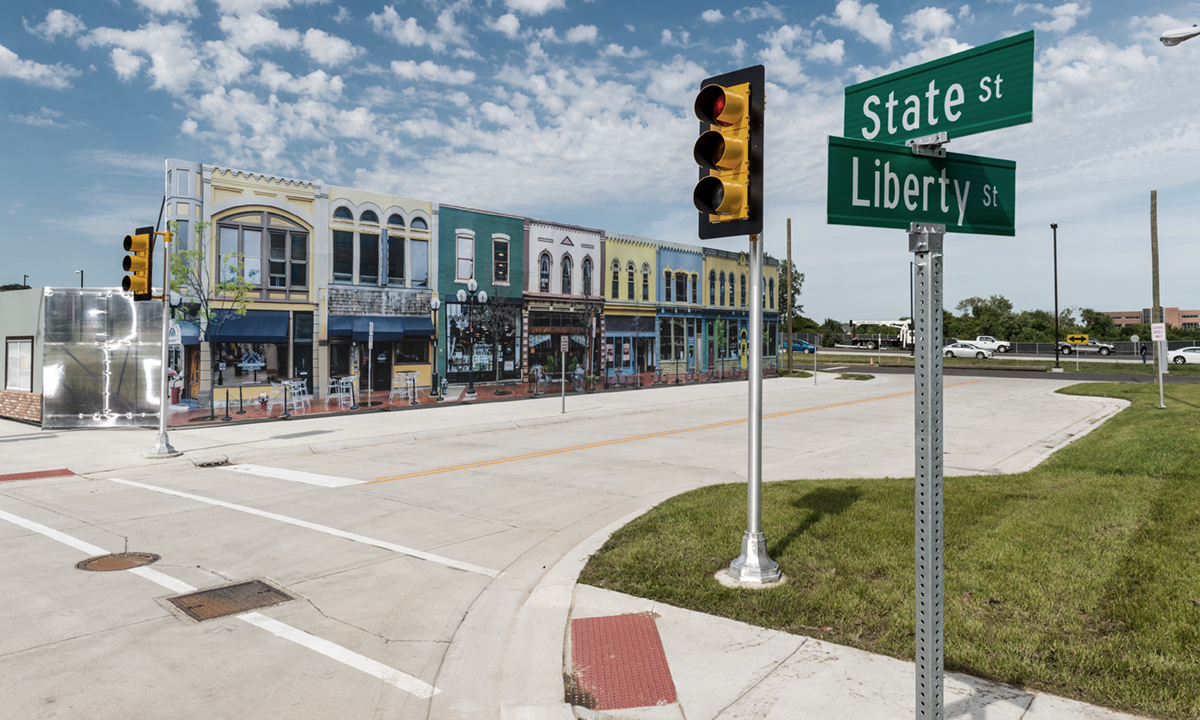
Simulated city streets at Mcity test the decision-making ability of automated vehicles. Photo credit: Mcity.
The key bottleneck is technology, but what specifically is the challenge?
It’s really the safety, and that challenge comes in two dimensions.
The first I call the “curse of dimensionality.” The current driving environment for human drivers is very complex. That complexity comes from a number of dimensions like weather conditions and different road infrastructures, like traffic lights or signs unique to a certain location. And then you have different road users, like pedestrians and cyclists, who each have different behaviors, like how older drivers tend to drive slow while younger drivers are the opposite. Machine learning models are solving that complexity, but they aren’t quite there yet. That’s because of the second challenge, which I call the “curse of rarity.”
This curse of rarity – or what can be called “corner cases” – lies atop the curse of dimensionality. Autonomous vehicles can handle 99.99% of use cases. But once you get to the 0.001%, AVs may not be able to handle it because they haven’t seen the scenarios yet. The machine learning model isn’t trained for it.
Some mobility players deploy AVs on freeways because they think urban streets have too many of those corner cases, while others do the opposite because they want cities to be the first areas where AVs scale up. Where do you fall on that?
I see two approaches. One is that we need breakthroughs in machine learning so that models can handle these corner cases. The second approach is to reduce the number of corner cases significantly. Some prefer freeways because it’s cleaner and have fewer the corner cases than urban streets.
In terms of reducing corner cases, many are wondering if we can provide information using not only onboard sensors but also roadside sensors. Maybe that will deliver information earlier,
so we don’t have corner cases – the machines know the cases before they encounter them. We need to provide AVs with information from the streets. For that, you need connectivity infrastructure to bridge that communication.
If a mobility service provider runs a fleet of AVs and provides that service to users, they can dispatch their vehicles to certain routes that can provide infrastructure support for AV operations.
Roadside communication requires some sort of data platform that everybody agrees on. How much have we matured toward a standard that different global players can resort to?
There are two key issues that still remain before that symbiosis can mature, and the first is establishing trust between AVs and roadside sensors. Can AVs trust their accuracy all the time? When there’s a certain object in certain places, what’s the confidence in that accuracy? That’s the number one goal for our smart intersection project.
Another issue is latency. How soon can you get the data to the AV? And is that data still fresh enough for the AV to use by the time it’s delivered?
If the endgame requires connected infrastructure, then we’re talking a much longer period of time to actually get to mass production. If we rely on AV sensors only, it could happen much faster – and a lot of players in this field prefer a vehicle-centric approach. Will there be a vehicle-centric approach or a cooperative, hybrid approach for the mass production of AVs?
Both methods are being developed, and I see potential for both. The vehicle-centric approach is more elegant. There’s less complexity, and it’s more scalable. But can that approach overcome the curse of rarity? The connected infrastructure approach is more complex, and many problems may be introduced – for example, the connectivity issue we discussed before.
Most likely some sort of combination will emerge – one in which AVs are supported by infrastructure in complex driving situations but rely on onboard systems only in simple scenarios.